J. Northw. Atl. Fish. Sci., Vol. 55: 01-10
Lindsey Noel Nelson1, 2,*
, W. David McElroy3, Andrew W. Jones2, George A. Maynard3
1Earth Resources Technology, Inc., 14401 Sweitzer Lane, Suite 300,
Laurel, MD 20707, United States of America
2NOAA Fisheries, Northeast Fisheries Science Center, Cooperative Research Branch,
28 Tarzwell Dr., Narragansett, RI 02882, United States of America
3NOAA Fisheries, Northeast Fisheries Science Center, Cooperative Research Branch,
166 Water St, Woods Hole, MA 02543, United States of America
*Corresponding Author: lindsey.nelson@noaa.gov (Lindsey Noel Nelson)
Nelson, L.N., McElroy, W.D., Jones, A.W., and Maynard, G.A. 2024. How the collection of fish length data using electronic monitoring video review measures up to current methods used on a fishery-independent survey. J. Northw. Atl. Fish. Sci., 55: 1–10. https://doi.org/10.2960/J.v55.m746
Abstract
Electronic monitoring (EM) systems are tools that can generate fisheries survey data when at-sea challenges such as on-deck logistics, workload capacity, or deployment interruptions prevent staff from fulfilling their duties. We sought to validate EM’s specific utility in collecting fish lengths at a comparable resolution to those collected at sea during a fishery-independent survey, the Gulf of Maine Bottom Longline Survey. We also examined whether measurement accuracy was influenced by tail morphology and length type (fork, total, and stretched total) by selecting individuals from six anatomically variable species. Individuals were measured twice: Survey-based length measurements, LS, were recorded using an electronic measuring board and EM-based length measurements, LE, were visually estimated using a color-coded EM measuring strip during video review. Paired Wilcoxon signed rank tests determined significant differences between the LS and LE E distributions for all species overall, and for individual species Atlantic cod, cusk, haddock, and spiny dogfish but not thorny skate or white hake. Kolmogorov-Smirnov tests detected no difference between the distributions of LS and LE, overall and for each species. Examination of the differences between LS and LE for every individual, LD, indicated that the EM-based method slightly over-estimated lengths (μ = 0.89 mm). Linear regression indicated that the effect of extreme small or great lengths on absolute LD was present only for Atlantic cod where LD increased as fish length decreased. Pairwise comparisons of LD among fish length types indicated that fork and stretched total length measurements were overestimated by the EM-based method (μ = 2.39 mm, 3.09 mm, respectively) and this was significantly more than total length (μ = 0.04 mm). We demonstrated that collection of fish lengths using video review could be an adequate substitution for collecting lengths by hand, though it is at the discretion of the end users to determine whether these length differences exceed the acceptable range. These results have particular applications to small scale survey operations, research, and the fishery-dependent sector.
Keywords: fisheries management, Gulf of Maine, fish morphology, subsample analyses
PDF & Supplementary Material
Download Citation Data

Citation to clipboard
Reference management software (Endnote, Mendeley, RefWords, Zotero & most other reference management software)

LaTex, BibDesk & other specific software
Introduction
Over the last two decades, electronic monitoring (EM) systems have been increasingly implemented in worldwide fishery-dependent data collection and fisheries management (van Helmond et al., 2020). These systems tend to consist of a central control unit, GPS, mechanical sensors, and video cameras that record footage of fishing activity (McElderry, 2008). The resulting video can be reviewed to collect fishery-dependent data, supplementing or replacing on-board observers. EM systems may be preferable to on-board observers when a vessel’s size or configuration is not suited to host an additional person, when there is a need for simultaneous viewing of multiple angles of activity, or when observation periods extend beyond reasonable human capabilities. EM systems are also deployed to validate fishing location and duration, identify protected species interactions, and document regulatory compliance.
Fishery-independent surveys present a unique platform for experimental testing, but challenges around increased staff workload or staff deployment exist. Camera systems can solve these problems by generating a means of post-survey data collection, and they have been successfully deployed alongside traditional fisheries survey gear (Starr et al., 2016; Williams et al., 2016; Stokesbury et al., 2017; Miller et al., 2019; Lee et al., 2022; Nelson et al., 2024). The crossover applications between survey and industry-based operations include exploration of technological limits, validation of new applications, and compilation of annotated video or photographic data for advanced machine learning and automated video processing.
The Gulf of Maine Bottom Longline Survey (BLLS) is a twice yearly, cooperative groundfish survey conducted on contracted commercial fishing vessels by the U.S. Northeast Fisheries Science Center (NEFSC). Beginning in 2020, camera systems were deployed on the vessels to test their potential utility in survey research activities (Nelson et al., 2024). The present study seeks to validate the use of EM as a method for collecting fish lengths that are comparable to lengths collected via more traditional methods used on fisheries-independent surveys. Similar validation studies have been conducted in the Taiwanese longline albacore tuna fishery (Chang et al., 2010), Welsh lobster and brown crab fisheries (Hold et al., 2015), the Pacific halibut longline fishery (Wallace et al., 2015), and Solomon Islands longline fisheries (Hosken et al., 2016). Here, lengths were recorded twice from individuals of six anatomically variable groundfish species according to their specified measuring methods: the first measurement using at-sea data collection protocols and the second using EM protocols. The resulting measurement distributions were compared and the calculated differences between each measurement method were further examined.
Materials and Methods
Fish Measurement Data Collection
Field surveys were conducted in accordance with survey protocols (McElroy et al., 2019) on the contracted partner vessel F/V Mary Elizabeth. The vessel crew members were responsible for setting and retrieving the longline gear and the NEFSC survey staff collected catch and biological information. The AI Hub and SnapIT camera system were provided and installed by the electronic monitoring service provider Teem Fish Monitoring of Prince Rupert, British Columbia, Canada. A camera with 720p high definition resolution (1920x1080) and a frame rate of 30 frames per second was mounted underneath the deck overhang and aimed directly down and over the processing table. Video of catch processing was continuously recorded onto a hard drive throughout each trip. Following completion of the survey, video was processed, uploaded to cloud storage, and viewed using the proprietary software provided by Teem Fish Monitoring.
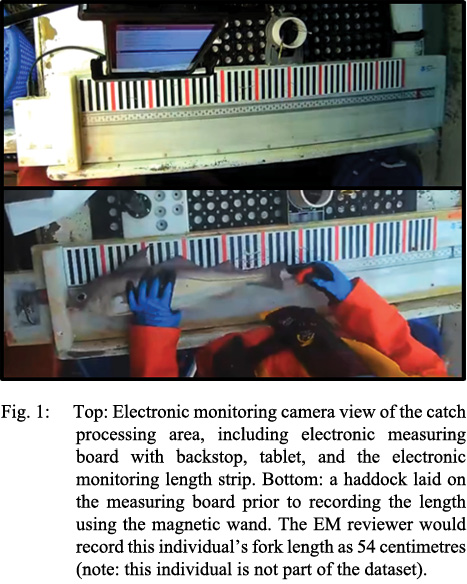
Fish length data collected during the survey, hereby referred to as LS, were recorded to the nearest millimetre with the Ichthystick II Electronic Fish Measuring Board (Price et al., 2015). The board consists of an electronic measuring stick with visible tick marks housed in a rectangular plastic case and backstop at the 0 centimetre mark (Fig. 1). When calibrated properly, the accuracy of the Ichthystick II is ±0.1 centimetres with a slight negative bias. Fish were laid on the measuring board as flat as possible with the snout against the backstop. Measurements were taken by tapping a magnetic wand onto the plastic housing at the desired measurement location. Measurements were automatically saved into a tablet-based data collection application. Lengths collected using EM protocols, hereby referred to as LE, were collected using a color-coded EM measuring strip overlaid on the electronic measuring board, also provided by Teem Fish Monitoring. Post-survey video review and collection of LE was conducted by a NEFSC staff member. When a candidate fish was held steady on the measuring board, the video was paused and fish lengths were visually determined by the reviewer using the EM measuring strip markings, consistent with the NEFSC Northeast Fisheries Monitoring Operations Branch guidance (Pers. Comm. Corinne Endres). To reduce bias, the moment in the video when LE was collected may not have been the exact moment when LS was collected. The design of this length strip consisted of colored markings in 1-centimetre increments (Fig. 1). The length of 0.01–1.00 centimetres was recorded as 1 centimetre, 1.01–2.00 centimetres was recorded as 2 centimetres, etc.; therefore, fish lengths were recorded as whole centimetres. Marking color aided in quick assessment of length: white markings were odd values, black markings were even values, and red markings were every 10 centimetres. Records of LS and LE as well as station data were merged into one dataset in R Studio version 1.4.1106 (R Core Team 2021, RStudio Team 2021). LE were converted from centimetres to millimetres for direct comparison to LS and reduced by 5 mm. This reduction shifts LE records around the center of each 1 cm marking and conforms to traditional rounding convention. For example, a fish that fell on a black bar between 41.01 and 42.00 cm was initially recorded as 42 cm, then converted to 420 mm and reduced to 415 mm. LS are the reference measurements and were not adjusted in any way.
We also examined whether morphology and length type impacted the accuracy between LS and LE. To do this, we selected the following six fish species with diverse tail morphologies that are also well represented in the BLLS: Atlantic cod (Gadus morhua), cusk (Brosme brosme), spiny dogfish (Squalus acanthias), haddock (Melanogrammus aeglefinus), white hake (Urophycis tenuis), and thorny skate (Amblyraja radiata). The length type used for each species is based on the NEFSC bottom trawl survey’s protocols. Total length, recorded as the measurement from tip of the snout to the tip of the tail, was recorded for species with rounded tails (Atlantic cod, cusk, and white hake) or pointed tails (thorny skates). Fork length, recorded as the measurement from tip of the snout to the deepest part of the tail fork, was recorded for the only species with a slightly concave tail (haddock). Finally, stretched total length, recorded as the measurement from tip of the snout to tip of the upper tail lobe when the tail was stretched down and in line with the body midline, was recorded for the only species with a forked tail with asymmetric lobes (spiny dogfish).
Identifying subset of stations and individuals
The dataset included individuals from three sampling periods: spring (April–May) 2021 and 2022, and autumn (October–November) 2021. LS was recorded for all catch encountered on the survey, but recording a census LE from all individuals of the chosen species would have been very time consuming. Therefore, for the purposes of this study and for each species, a representative subsample of individuals from the BLLS population was identified. We estimated the target subsample size for each species using two methods. First, the R package “samplingbook” version 1.2.4 (Manitz et al., 2021) was used to estimate subsample sizes with the same mean length ±5%, standard deviation, and a 95% confidence interval as the BLLS population. Second, Kolmogorov-Smirnov (K-S) tests determined the minimum subsample size needed to produce a similar distribution to the BLLS population (Marsaglia et al., 2003). To determine the appropriate sample size, a simulation of fish length resampling without replacement from the BLLS population was conducted for subsample sizes from 10 to 100 by intervals of 10 and a K-S test was conducted on each subsample by calling the ks.test command from the package “stats” version 4.2.2. This process was repeated for 1,000 iterations and the resulting plots of K-S test p-values were used to visually determine a target subsample size (Supplementary Material S1). The smallest subsample size per species in which the p-values of all 1,000 iterations was above 0.05 was selected as the minimum subsample size. The plots for the three most abundant species (cusk, spiny dogfish, and haddock) did not indicate any differentiation among subsample sizes, so sample sizes of 50 were arbitrarily selected for the K-S method. The greater of the two subsample estimation methods was selected as a minimum subsample size target for each species (Atlantic cod = 72, cusk = 50, haddock = 50, spiny dogfish = 50, thorny skate = 87, and white hake = 50; Supplementary Material S2).
Next, we identified a subset of candidate stations from which to measure all available individuals of the interest species. From the initial 69 stations across the three survey cruises, only 64 of those had length data available at the time of the data pull. We eliminated four stations where the video was available (i.e. not missing due to power failure or system malfunction), 4 stations where the length strip was missing from the measuring board, and one station where the length strip was improperly placed. For each distinct species, the remaining 55 candidate stations were further reduced according to species specific length thresholds in order to avoid cluster sampling of the most common lengths. Each available station must have at least one representative below the lower length threshold or above the higher length threshold (Supplementary Material S2, S3). The thresholds for Atlantic cod, cusk, spiny dogfish, and white hake were the median length ±50%, while the threshold for thorny skate was the median length ±20%, due to its non-normal length distribution. The stations were finally narrowed according to a minimum and/or maximum number of individuals measured at that station. These limits were different for each species and dependent on whether the species was typically caught as schools or as incidental individuals. Prioritizing stations with the greatest number of individuals increased our data collection efficiency. The resulting candidate stations were visually assessed and selected such that the full length-range of each species was represented and the minimum subsample size was achieved.
Analysis
Analyses comparing the LS and LE distributions were conducted for all species combined and each species separately. The first comparison was the Wilcoxon signed rank test, a non-parametric test of equal means between paired samples which does not assume equal variance, by calling the wilcox.test command and specifying paired = T from the R package “stats” version 4.2.2 (Wilcoxon, 1945; Bauer, 1972). The second comparison were K-S tests as described previously. Mathematical differences between the survey and EM data collection methods, hereby referred to as LD, were calculated for each individual where LD + LE - - LS This value evaluates whether the EM method over- or under-estimated length measurements (positive or negative LD values, respectively). Records with LD >±10 millimetres were reevaluated in video review, taking care to note circumstances surrounding their measurement and possible sources of error such as measuring incorrect individuals, fish handling, fish condition, EM measuring strip position, and view obstructions. From these records, there were two instances where the EM measuring strip had shifted away from the measuring board backstop, creating offsets of 10 and 40 millimetres. The LE for these records were corrected by adding the number of millimetres associated with the specific offset. An additional test to determine whether absolute LD values correlated with fish length or whether any species was prone to increased LD was conducted by calling the lm command from the R package “stats” version 4.2.2, where absolute LD was predicted as a function of LS. Finally, comparisons of mean LD among length types (fork, total, stretched total) were made using t-tests.
Results
A total of 388 fish were included in the dataset. For each species, the number of individuals (n), the length range, and mean length (μ) were as follows: Atlantic cod (n = 74, 246–759 mm, μ = 480.28 mm), cusk (n = 55, 321–746 mm, μ = 514.26 mm), haddock (n = 56, 269–628 mm, μ = 444.04 mm), spiny dogfish (n = 65, 443–919 mm, μ = 739.75 mm), thorny skate (n = 84, 382–959 mm, μ = 537.39 mm), and white hake (n = 54 356 –888 mm, μ = 618.50 mm). For all species collectively, differences between the LS and LE distributions were detected by the paired Wilcoxon test (μ = 554.94 mm and 560.82 mm, respectively; p 0.001) but not the K-S test (Fig. 2, Table 1). When comparing LS and LE for each species, the paired Wilcoxon test detected differences for Atlantic cod, cusk, haddock, and spiny dogfish (p < 0.001) but again, the K-S tests did not detect any statistical differences between the distributions.
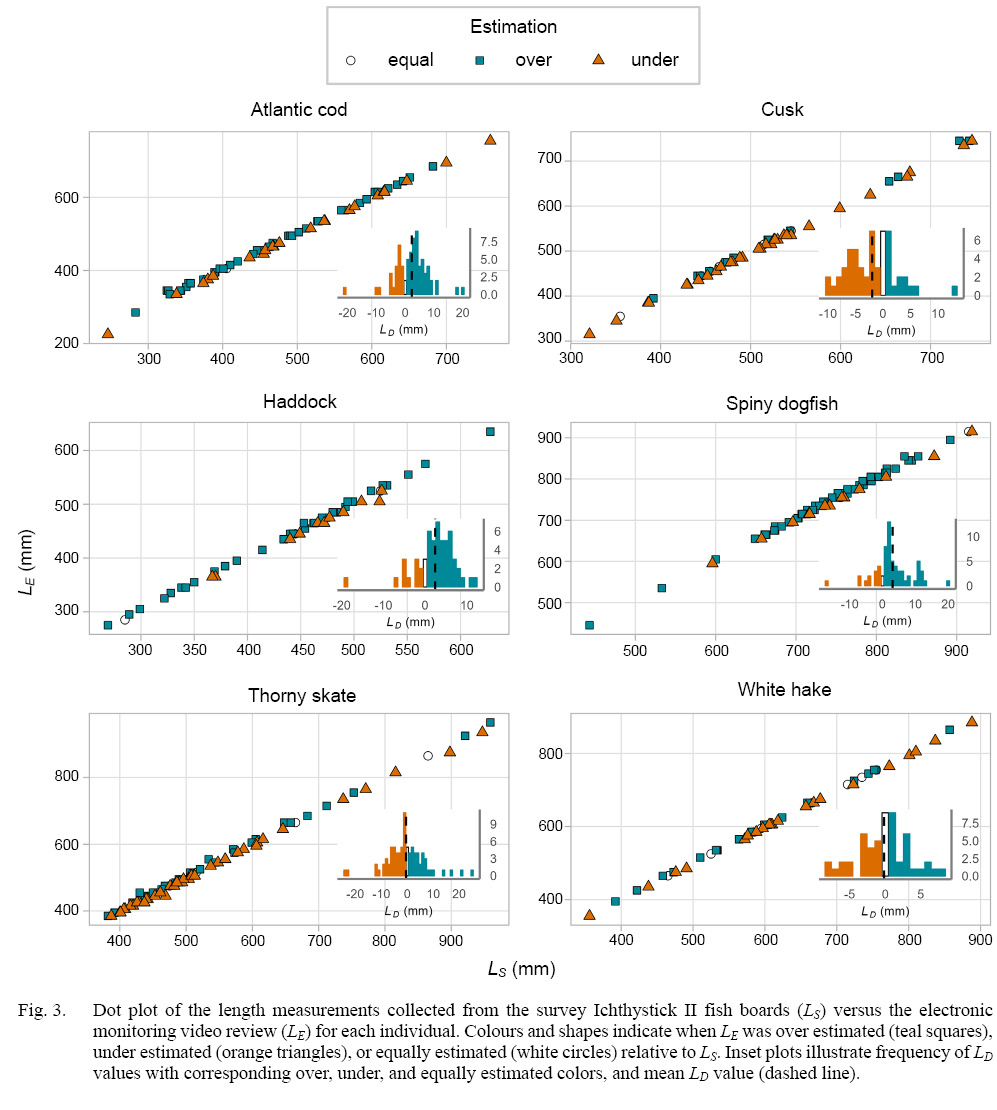
The mean LD values provided evidence for length over-estimation by the EM measurement method for Atlantic cod, haddock, and spiny dogfish, and evidence for under-estimation for cusk, thorny skate, and white hake (Fig. 3, Table 1). Regardless of the direction, these values were very small (-1.98–3.09 mm, overall μ = 0.89 mm). White hake had the lowest LD range and standard deviation (-8–8 mm, 3.55 mm, respectively) and thorny skate had the greatest LD range and standard deviation (-24–25 mm, 7.48 mm, respectively), the latter indicated both a greater frequency and magnitude of non-zero LD. Linear regression did not detect a significant relationship between LS and absolute value of LD for all fish collectively, meaning that in general, individuals of extreme small or large lengths are not prone to increased absolute value of LD. Of the individual species, only Atlantic cod exhibited a significant trend, where absolute value LD was highest at small lengths and decreases with increasing fish length (β = -0.013, p = 0.003).
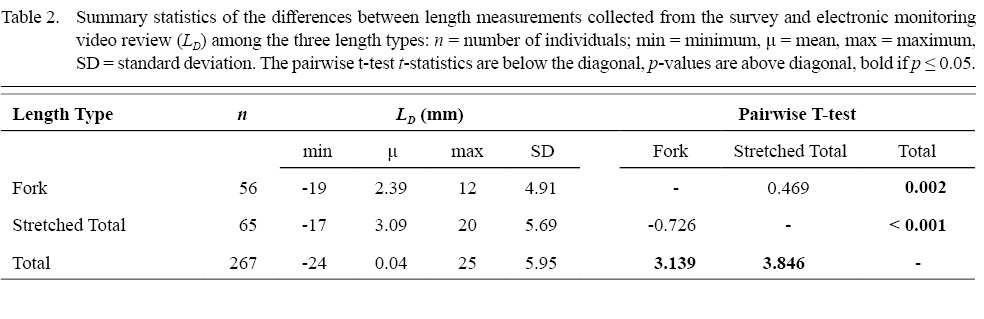
Among the three length types, total length had near-zero mean LD and the highest standard deviation (Fig. 4, Table 2). Fork length and stretched total length had comparatively higher mean LD, and lower standard deviations. Pairwise comparisons indicated that the differences in mean LD were significantly different between total and both fork and stretched total length (p = 0.002, p 0.001, respectively) and that there was no difference between the mean LD of the latter two (p = 0.469). Because both fork and stretched total length were comprised of a single species and total length was comprised of four species the resulting uneven sample sizes among the measurement methods may have introduced bias in the calculations.
Discussion
This study successfully demonstrated that EM systems and associated video analysis can be used as a tool to collect fish lengths at an accuracy comparable to the lengths collected by fishery-independent longline survey staff. Depending on the test used, the distributions of LS and LE may be statistically different from each other. Disparities were most evident when examining LD, the mathematical differences between LS and LE. The EM method over-estimated lengths for half of our species and under-estimated for the other half. Although some of these distributions were statistically different, the effect on downstream BLLS data end users would be minimal in practice. However, this may not be the case for other data collectors and users, and the precision of the data should be considered in the context of individual programs.
The primary source of non-zero LD stems from the study design itself. Comparing measurements from two units of measure (centimetres vs millimetres) inherently loses precision and the chances that the reading of one 1-cm mark matches the reading from the 1-mm unit board is not common. A second source of non-zero LD stemmed from fish handling and positioning on the EM measuring strip. Despite best efforts to lay fish flat, lively individuals defensively and evasively curled or twisted themselves. A change in the position or configuration of an individual between the times when lengths were recorded on the BLLS and recorded from the video could have affected measurement accuracy. For example, second review of the video for the greatest LD record for Atlantic cod (-21 mm) revealed that the fish curled its tail upward, towards the camera, though at first glance, it appeared to be resting flat. To mitigate this source of increased LD, the EM reviewer must not rush data collection and ensure the fish is truly laid flat. A third source of non-zero LD was the improper alignment of the EM measuring strip on top of the electronic measuring board and against the backstop. This occurred for two records of thorny skate, as noted prior. In the event the EM measuring strip becomes offset for an entire haul or basket of fish, a batch of consistently negative LD would be evident and should be regularly checked for in data quality procedures. To mediate improper alignment between the EM measuring strip and the backstop we recommend using a fixed or permanently integrated EM measuring strip.
It is important that studies using EM should consider two potential sources of non-zero LD that were not directly observed here. The first is the distortion of the measuring strip at the edges of the camera view. In the current study, the camera was centered over the measuring board backstop and the lower range of measurement values. As the EM measuring strip extended away from the center of the camera view, the 1-centimetre wide markings narrowed, and this distortion presents an opportunity to introduce uncertainty. To minimize this, efforts should be made to center the camera over the EM measuring strip and install the camera at a distance from the EM measuring strip that would reduce image distortion. A second source of potential LD may be in the accuracy of the measurement tools. The level of error for the Ichthystick II boards is low (±0.1 cm) and well within the nearest whole or half centimetre data standards required by most of the U.S. Northeast region stock assessments (Grosslein, 1969;Wigley et al., 2003; Price et al., 2015). The standard deviations of LD reported here also fall within the whole centimetre data standard, which support the idea that lengths collected by EM systems could be a valid component of stock assessments. The design of the particular EM measuring strip is also important to note. Providers of EM systems supply their own independently designed measuring strips for regulatory compliance. The measuring strip and subsequent initial measuring method in this study did not conform to traditional rounding standards, thus necessitated shifting LE by 5 mm. Proper rounding is a critical component to estimating abundance of year classes or when dealing with harvest size limits. The accuracy of EM-collected lengths using alternative EM measuring strip designs should be tested for by conducting studies in a similar manner to the present one.
We detected differences in measurement accuracy among fish length types. Morphological diversity prevents a single length type from being used for all species, but standardized measures based on shared morphology are commonly used (Francis, 2006). These standardized measures can be of varying reproducibility success and therefore potentially introduce variability into LD. For example, a study of three freshwater species found lower mean and standard deviation for fork length and total length compared to “standard length”, a length type that has a history of inconsistent definition, for three freshwater fish species (Carlander and Smith Jr., 1945). Furthermore, fork length may be more easily reproducible than standard length or total length, though the difference in accuracy is marginal (Kahn et al., 2004). Although the handling of fish while collecting fork length and total length in the BLLS are similar (i.e. no manipulation of tail placement), the distributions of LD were statistically different between them. The greater variance seen in total length in this study may be driven by the data from thorny skates, which have the highest variance of all examined species. It was expected that stretched total length would have the highest variance because the extent to which the anterior tail lobe is stretched by the handler to the center of the measuring board may not be consistent. In addition, spiny dogfish are notoriously lively and their bending and flexing could create measurement inconsistencies that are independent of the stretched length methodology. The results among length types may have been further influenced by unequal sample sizes, which could be clarified by conducting the study again with equal number of fish per length type or with the inclusion of other species with slight morphological differences.
This study was performed by collecting one LE per fish by a single video reviewer. Although between-reviewer variability is routinely assessed when estimating fish ages (Campana et al., 1995; Buckmeier, 2002), few such comparisons exist for morphological measurements (Petrtýl et al., 2014). Additional investigation of between-reviewer variability would provide insight into the feasibility and caveats of collecting length data using EM, expansion towards team-oriented data collection, development of data collection protocols, and conducting routine between-reader tests. Further, it is important to note that EM video reviewers should follow fish measuring protocols according to guidelines set by data users, thereby minimizing measurement variation among datasets and the time spent creating protocols or adjustment factors.
The current study demonstrated how camera system utilization can increase the volume of scientific data and expand survey operations. The camera system and length strip equipment simplify fish length measurement collection process such that proper placement and handling of the fish in front of the camera can be performed by a single person with minimal training. In addition, a durable length strip and post-survey data collection eliminates the onus of collecting or transcribing data by hand, maintaining water sensitive cables and costly tablets, and troubleshooting Bluetooth connectivity issues, as are encountered on the BLLS. These benefits are particularly applicable to small or industry vessels, where installation of EM systems or recruitment of those with existing systems can open doors for collaborative projects with government agencies and academic institutions (Sigler and Lunsford, 2009; McElroy et al., 2019). Such collaborative efforts have gained support in recent years. For example, offshore wind development in the United States Northeast region will impact existing federally based surveys (Methratta et al., 2023), and smaller industry based surveys are being looked to as alternatives. Similarly, the Gulf of Maine shrimp fishery participants are responding to federal funding cuts to the current sampling program by mobilizing self-funded and operated sampling efforts, part of which involves EM camera equipment (Molyneaux, 2024). The benefits of using EM to collect fish lengths is less applicable to mature, large scale fishery-independent surveys. In these survey operations, collection of fish lengths is a regular task that can account for a large proportion of catch processing (Link et al., 2008) and replacing well-established, systematic methods with video-based and post-survey methods is unlikely to improve efficiency. However, EM could be a viable backup option in the event of scientific staffing shortages or increased reliance on vessel crew to collect data. There is also application of this work in the fishery-dependent sector. EM review could serve as an oversight tool where catch is sorted into market categories or product types based on fish length, such as at processing plants or on catcher-processor vessels. It could also be an effective way to collect lengths at any size range, expanding its use from discards to retained catch lengths. This last point is particularly useful as funding for port samplers or on-board observers is reduced or not keeping pace with the required cost.
Acknowledgements
We extend our gratitude to the captain of the Mary Elizabeth, Captain Phil Lynch, and his crew, including Steve Kenney, for accommodating our technological and data collection needs. Special thanks to the staff at Teem Fish Monitoring for technical assistance with camera system hardware installation and training on the Engagement software. We acknowledge NOAA staff Jessica Blaylock, Nichole Rossi, and Corinne Endres for sharing their expertise, as well as Lisa Peterson for reviewing the manuscript. And finally to the reviewers and editors of the Journal of Northwest Atlantic Fishery Science, whose suggestions greatly improved this manuscript.
References
Bauer, D. F. 1972. Constructing Confidence Sets Using Rank Statistics. Journal of the American Statistical Association, 67(339): 687–690. https://doi.org/10.2307/2284469.
Buckmeier, D. L. 2002. Assessment of reader accuracy and recommendations to reduce subjectivity in age estimation.Fisheries, 27(11): 10–14. https://doi.org/10.1577/1548-8446(2002)027<0010:AORAAR>2.0.CO;2
Campana, S. E., Annand, M. C. and McMillan, J. I. 1995. Graphical and Statistical Methods for Determining the Consistency of Age Determinations. Transactions of the American Fisheries Society, 124(1): 131–138. https://doi.org/10.1577/1548-8659(1995)124<0131:GASMFD>2.3.CO;2
Carlander, K. D., and Smith, L. L. Jr. 1945. Some Factors to Consider in the Choice between Standard, Fork, or Total Lengths in Fishery Investigations. Copeia, 1945(1): 7–12. https://doi.org/10.2307/1438165.
Chang, S.-K., DiNardo, G., and T.-T. Lin. 2010. Photo-based approach as an alternative method for collection of albacore (Thunnus alalunga) length frequency from longline vessels. Fisheries Research, 105(3): 148–155. https://doi.org/10.1016/j.fishres.2010.03.021.
Francis, M. P. 2006. Morphometric minefields—towards a measurement standard for chondrichthyan fishes.Environmental Biology of Fishes, 77(3-4): 407–421. https://doi.org/10.1007/s10641-006-9109-1.
Grosslein, M. D. 1969. Groundfish survey methods. Lab Ref Num 69-2.
Hold, N., Murray, L. G., Pantin, J. R., Haig, J. A., Hinz, H. and Kaiser, M. J. 2015. Video capture of crustacean fisheries data as an alternative to on-board observers. ICES Journal of Marine Science, 72(6): 1811–1821. https://doi.org/10.1093/icesjms/fsv030.
Hosken, M., Vilia, H., Agi, J., Williams, P., Mckechnie, S., Mallet, D., Honiwala, E., Walton, H., Owens, M., Wickham, C., Zaborovskiy, E., and Cheung, B. 2016. Report on the 2014 Solomon Islands Longline E-Monitoring Project.
Kahn, R. G., Pearson, D. E. and Dick, E. J. 2004. Comparison of Standard Length, Fork Length, and Total Length for Measuring West Coast Marine Fishes. Marine Fisheries Review. 66(1).
Lee, Y.-D., Lee, H., Yoon, E., Park, C., Osborg, E. S., and Løvall, K. 2022. A Comparative Assessment of Mid-Water Trawl and Deep Vision for Investigating Fishery Resources in the Coastal Waters off Jeju Island, Korea. Applied Sciences. 12(4).
Link, J., Burnett, J., Kostovick, P. and Galbraith, J. 2008. Value-added sampling for fishery-independent surveys: Don’t stop after you’re done counting and measuring. Fisheries Research, 93(1-2): 229–233. https://doi.org/10.1016/j.fishres.2008.04.011.
Manitz, J., Hempelmann, M., Kauermann G., Kuechenhoff, H., Shao, S., Oberhauser, C., Westerheide, N., and Wisenfarth, M. 2021. samplingbook: Survey Sampling Procedures. R package version 1.2.4, https://CRAN.R-project.org/package=samplingbook.
Marsaglia, G., Tsang W. W., and Wang, J. 2003. Evaluating Kolmogorov's Distribution. Journal of Statistical Software, 8(18): 1–4. https://doi.org/10.18637/jss.v008.i18.
McElderry, H. 2008. At-Sea Observing Using Video-Based Electronic Monitoring. Background paper prepared by Archipelago Marine Research Ltd. for the Electronic Monitoring Workshop.
McElroy, W. D., O’Brien, L., Blaylock, J., Martin, M. H., Rago, P. J., Hoey, J. J. and Sheremet, V. A. 2019. Design, Implementation, and Results of a Cooperative Research Gulf of Maine Longline Survey, 2014–2017. NOAA Technical Memorandum NMFS-NE-249. US Department of Commerce, National Oceanic & Atmospheric Administration, National Marine Fisheries Service, Northeast Fisheries Science Center.
Methratta, E. T., Silva, A. Lipsky, A., Ford, K., Christel, D., and Pfeiffer, L. 2023. Science Priorities for Offshore Wind and Fisheries Research in the Northeast U.S. Continental Shelf Ecosystem: Perspectives from Scientists at the National Marine Fisheries Service. Marine and Coastal Fisheries. 15(3). https://doi.org/10.1002/mcf2.10242.
Miller, T. J., Hart, D. R., Hopkins, K., Vine, N. H., Taylor, R., York, A. D. and Gallager, S. M. 2019. Estimation of the capture efficiency and abundance of Atlantic sea scallops (Placopecten magellanicus) from paired photographic–dredge tows using hierarchical models. Canadian Journal of Fisheries and Aquatic Sciences, 76(6): 847–855. https://doi.org/10.1139/cjfas-2018-0024.
Molyneaux, P. (2024). Fishermen hope to find Gulf of Maine shrimp stocks revived. National Fisherman.
Nelson, L. N., McElroy, W. D., Jones, A. W., Maynard, G. A., Tholke, C., and Mercer, A. J. M. 2024. Taking the bait: Environmental factors affecting bait retention and hook disposition in the Gulf of Maine Bottom Longline Survey. Fisheries Research. 273. https://doi.org/10.1016/j.fishres.2024.106957.
Petrtýl, M., Kalous, L. and Memİş, D. 2014. Comparison of manual measurements and computer-assisted image analysis in fish morphometry. Turkish Journal of Veterinary and Animal Sciences. 38: 88–94. https://doi.org/10.3906/vet-1209-9.
Price, V., Godlewski, J., Jech, J. M. and McHugh, N. 2015. Calibration of Electronic Measurement Boards and Length Values Recorded in the Fisheries Scientific Computing System (FSCS). NOAA technical memorandum NMFS-NE-232. US Department of Commerce, National Oceanic & Atmospheric Administration, National Marine Fisheries Service, Northeast Fisheries Science Center.
R Core Team. 2021. R: A Language and Environment for Statistical Computing. https://www.R-project.org/.
RStudio Team. 2021. RStudio: Integrated Development Environment for R. http://www.rstudio.com/.
Sigler, M., and Lunsford, C. 2009. Survey protocol for the Alaska sablefish longline survey. Technical report. A. F. S. Center.
Starr, R. M., Gleason, M. G., Marks, C. I., Kline, D., Rienecke, S., Denney, C., Tagini, A., and Field, J. C. 2016. Targeting Abundant Fish Stocks while Avoiding Overfished Species: Video and Fishing Surveys to Inform Management after Long-Term Fishery Closures. PLoS ONE, 11(12): e0168645. https://doi.org/10.1371/journal.pone.0168645.
Stokesbury, K. D. E., Cadrin, S. X., Calabrese, N., Keiley, E., Lowery, T. M., Rothschild, B. J., and DeCelles, G. R. 2017. Towards an Improved System for Sampling New England Groundfish Using Video Technology. Fisheries, 42(8): 432–439. https://doi.org/10.1080/03632415.2017.1342630.
van Helmond, A. T. M., Mortensen, L. O., Plet-Hansen, K. S., Ulrich, C., Needle, C. L., Oesterwind, D., Kindt-Larsen, L., Catchpole, T., Mangi, S., Zimmermann, C., Olesen, H. J., Bailey, N., Bergsson, H., Dalskov, J., Elson, J., Hosken, M., Peterson, L., McElderry, H., Ruiz, J., Pierre, J. P., Dykstra, C., and Poos, J. J. 2020. Electronic monitoring in fisheries: Lessons from global experiences and future opportunities. Fish and Fisheries, 21(1): 162–189. https://doi.org/10.1111/faf.12425.
Wallace, F., Williams, K., Towler, R., and McGauley, K. 2015. Innovative Camera Applications for Electronic Monitoring. Fisheries Bycatch: Global Issues and Creative Solutions(eds.), Alaska Sea Grant, University of Alaska Fairbanks. https://doi.org/10.4027/fbgics.2015.06
Wigley, S. E., McBride, H. M. and McHugh, N. J. 2003. Length-Weight Relationships for 74 Fish Species Collected during NEFSC Research Vessel Bottom Trawl Surveys, 1992–99. NOAA Technical Memorandum NMFS-NE-171. US Department of Commerce, National Oceanic & Atmospheric Administration, National Marine Fisheries Service, Northeast Fisheries Science Center.
Wilcoxon, F. 1945. Individual Comparisons by Ranking Methods. Biometrics Bulletin. 1(6): 80–83. https://doi.org/10.2307/3001968.
Williams, K., Lauffenburger, N., Chuang, M.-C., Hwang, J.-N. and Towler, R. 2016. Automated measurements of fish within a trawl using stereo images from a Camera-Trawl device (CamTrawl). Methods in Oceanography. 17: 138–152. https://doi.org/10.1016/j.mio.2016.09.008.
Citation: Nelson, L.N., McElroy, W.D., Jones, A.W., and Maynard, G.A. 2024. How the collection of fish length data using electronic monitoring video review measures up to current methods used on a fishery-independent survey. J. Northw. Atl. Fish. Sci., 55: 1–10. https://doi.org/10.2960/J.v55.m746